
Well friends, we’re going to do things a little differently this week. For my standard introduction on what the PGA course fit model is, how it’s achieved, and how it helps us separate from the rest of the industry, I encourage you to revisit any of my past articles, including the course fit article for The Memorial.
I’m skipping the traditional introduction because this week, I want to highlight our newest addition, Josh Culp, and his excellent course notes and quotes. Specifically, I want to highlight how some seemingly contradictory analysis can not only coexist, but actually support one another. So, for this article, I’ll go category by category breaking down where the course fit model deviates, aligns, and builds off Josh’s analysis. Spoiler alert — there’s more in common than you’d initially think.
CF Model Results
Before we dive into the comparisons, a little about this week’s model. As you may have expected if you’re an avid reader, this week’s “course” fit model is actually a U.S. Open fit model. In other words, it’s built off players’ histories in the U.S. Open since we don’t have adequate data on The Country Club. For those who haven’t heard this spiel before, this type of analysis has three potential outcomes. We know the U.S. Open plays differently than other PGA Tour events, so a strong CF Model centered around tour average weights is a virtual impossibility, which leaves:
- A weak model, centered around tour averages
This result would indicate little continuity between how various U.S. Open courses challenge players, which in turn would mean that the CF Model would be unreliable for The Country Club.
- A weak model with unique weights
Similarly, this result would indicate severe fluctuation between one course and the next, with the presence of an overall bias in certain directions. The model would be unreliable, but not without value. If nothing else, it would point us to a viable skillset stack for certain lineups.
- A strong model with unique weights
Obviously, this is the result we’re hoping for. This result would indicate a consistency to how U.S. Open courses challenge players, indicating the signal is more about the USGA setup (or what they look for in a course they deem worthy of their championship) than individual characteristics about each course.
As you can see, every metric has a unique weight in the U.S. Open model, and the model as a whole is even more predictive than the PGA Championship, which yielded strong results. Boom, we’re off to a great start. So, how do the findings of the CF Model for the U.S. Open compare to Josh’s analysis?
Off the Tee
From Josh:
“A few years ago, we saw Bryson pick apart Winged Foot with the bomb-and-gouge approach, but I don’t see that being the smart route this week. Unlike Winged Foot, I do expect steady drivers to hit their fair share of these Brookline fairways, which will give them a leg up in the next category.”
Immediately, this seems contradictory. The CF Model points to distance being more important and accuracy less than important than usual, like at Winged Foot, but will it play out differently at The Country Club? Let’s revisit one of my favorite distinctions, between descriptive and predictive analysis:
- Descriptive: describes what has happened in the past
- Predictive: predicts what will happen in the future
I think Josh can be correct here without contradicting the CF Model because it will be difficult for a player to descriptively gain considerable strokes off the tee through distance without the presence of accuracy. However, that doesn’t mean distance can’t be more predictive. Perhaps my favorite example of this came in Cameron Young’s breakout performance at Riviera. On multiple occasions, he was hitting fairways with long irons where most of the field struggled to hit the same part of the fairway with 3-woods. In other words, his length was predictive of his descriptive accuracy since it allowed him to use a club that makes hitting fairways easier.
Personally, I think this week sets up as follows:
Short and accurate players will still miss fairways, and they’ll be at a tremendous disadvantage when they do. Long players will have a fighting chance when they miss fairways, so they can survive even if they’re rarely finding the short grass. They also may be able to use more accurate clubs when forced to club down, a-la Cameron Young at Riviera. Finally, long and accurate players will have a chance to experience compounding returns, where accuracy is rewarded disproportionately in the presence of distance than in the absence of it. Translation? Accuracy for short hitters can’t help much, but the long hitters who are also accurate could be in for a great week.
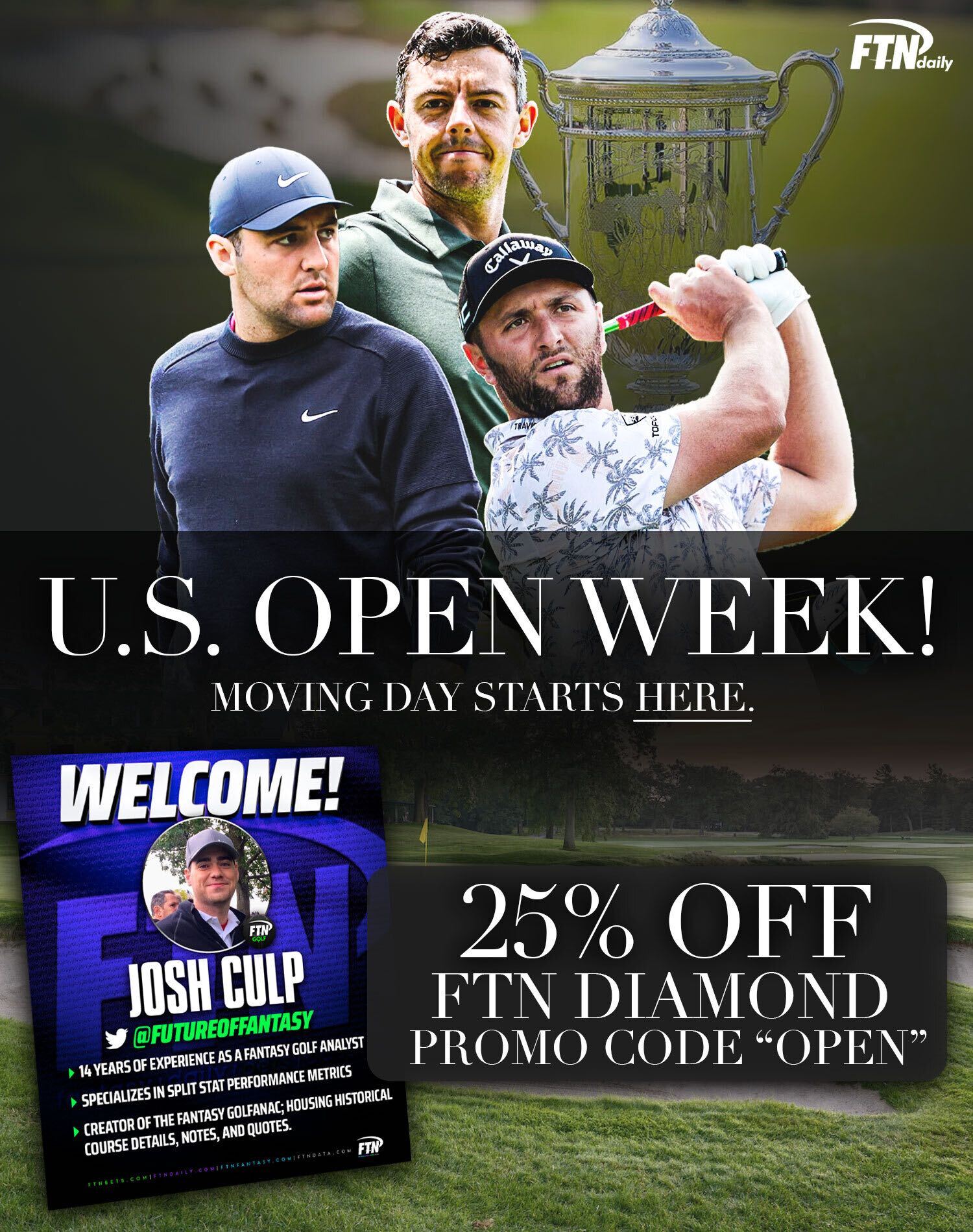
Approach
From Josh:
“Attacking from the fairway will likely be crucial, because the green targets are tiny (very tiny!) and well-protected by bunkers and false fronts.”
We are in complete agreement here. Approach play is far less predictive in U.S. Opens because a player’s opportunity to gain strokes with his irons is largely determined by his driving performance. Additionally, we likely see an effect similar to the one we saw at Bay Hill this year, where Rory McIlroy observed the fact that great iron shots weren’t always being rewarded.
Around the green
From Josh:
“I do think around-the-green form will be important, but I see that as more of a course-management indicator than actually needing to be elite chipping. Having a good game plan for the course and knowing where to miss is more important, since even the best scramblers will be unable to get up-and-down from some of the bad ATG lies this week.”
This is gold/ In fact, this is the section that gave me the idea to do the course fit article this way for the week. Here’s the thing — on the surface, it may seem like Josh is mitigating the predictive value of around-the-green play here, while the CF Model points to it being more predictive than usual. However, note that Josh’s point on course-management is always the case! Strokes-gained data is built solely off distance to the hole and the type of lie (i.e. fairway vs rough vs sand, etc.). Whether or not a player is short-sided, for example, is unknown to the SG calculations. Is it possible that that’s more true here at The Country Club than most tour events, or even most U.S. Opens? Of course, but I would bet that’s off-set by the increased frequency with which a player is forced to lean on his short game this week.
Conclusion? Josh’s point about short-game play stealing signal from overall course management is an awesome one, and one that helps explain the increased importance of ATG play in U.S. Opens. Long rough typically adds variance to short game play, which could theoretically make it harder for elite short-game players to consistently gain strokes around the green, so this explanation is a helpful one to mesh educated speculation with what the data tells us.
Putting
From Josh:
“The green sizes are small but very spicy! Kinda like Shakira. A lot of undulation in these. We know how the USGA likes to push it to the limits, so don’t be surprised if we see a repeat of Shinnecock over the weekend with Phil running across greens trying to hit it before it falls off the false front. Okay, I don’t expect to actually see that again, but my point is that I think putting will matter a lot despite the greens being so small.”
Ha — Josh took the long and comical route to saying exactly what the CF Model tells us: Putting matters in the U.S. Open. My best guess for why putting is so predictive is the fact that every player is going to be faced with a considerable number of testers left for par. This increased number of putts from more than gimme distance but less than hit-it-and-hope distance could decrease variance in overall putting performance.
Course Fit Specialists
OK, so who stands to benefit from these findings? Below is a list of players that I have more interest in due to their potential course fit than their baseline SG projections. This is not an exhaustive list of players I like, nor should it be considered a set of rankings. For more on exactly who I want to play this week, make sure you check out my Hot Takes article on Wednesday and as always, I’ll update my cheat sheets later in the week. Of course, you can also view all of the course fit and overall expected strokes gained projections, to see how your favorite players rate out this week.
Studs
Rory McIlroy
Cameron Smith
Jordan Spieth
Patrick Cantlay (no idea what to do with him right now)
Mid-Tier
Cameron Young (potential core play if he’s less popular than he’s been in most events recently)
Sam Burns (we play Burns even when he doesn’t project well so this has me all types of excited for Slammin’ Sammy)
Tyrrell Hatton
Tommy Fleetwood
Louis Oosthuizen
Values
Talor Gooch
Davis Riley
Patrick Reed
Alex Noren
Brian Harman
Shout out once again to Josh for his excellent FTN debut article, and let’s have ourselves a week.