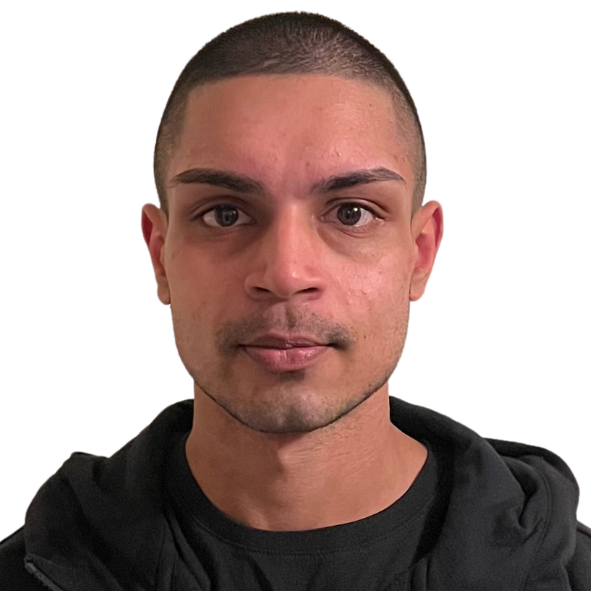
The word “correlation” gets tossed around in the fantasy sports and DFS pick ‘em worlds ad nauseam, but do you truly understand what it means? If you had to stand at the front of class tomorrow and talk about what it means and all its manifestations in these games we love so much, could you? By the end of this article, you’ll be ready to ace the hypothetical presentation with your chest held high.
There are three key components to understand:
- There are two types of correlation — direct and indirect, which we can also think of as obvious and hidden correlations.
- Correlation becomes more intuitive when you look at it through the lens of conditional probabilities.
- With the use of strong simulations, the power of correlation can be harnessed without even having to estimate the degree to which two or more things are correlated.
Direct and Indirect Correlation
Direct Correlation/Obvious Correlation
The first type of correlation is the obvious type — if Tyreek Hill blows by his yardage projection with over 200 receiving yards, it dramatically increases the chances that Tua Tagovailoa will go over 300 passing yards. If Freddie Freeman rips a double into the gap after a Mookie Betts double of his own, Freeman helps Betts score a run and Betts helps Freeman collect an RBI.
There was a time in the DFS pick ‘em world where even this correlation wasn’t guarded against, but the greatest things in life often come to an end, and now these sites have safeguards in place like decreased payouts to protect themselves from the correlation fiends (I am the correlation fiends). Is it possible to find instances where the sites are underestimating the correlation that exists between two players (or more)? Sure, but in all likelihood, we have to find edges elsewhere.
Indirect Correlation/Hidden Correlation
Here’s a typical flow chart to project production in a game for an NFL passing attack:
- Step 1 — project the number of plays
- Step 2 — project the pass rate
- Step 3 — project the overall pass efficiency
- Step 4 — project the target distribution
- Step 5 — project the individual receiving efficiencies
Now, suppose our models at FTN agreed on Steps 3-5 with the model powering Underdog’s projections for a game, but we projected a higher pass rate and a higher number of plays. More pass attempts with the same expected target distribution and efficiency for every individual player would mean our projections would be higher than Underdog’s. Consequently, we would show an edge on every player involved in the passing attack. This is fairly straightforward, but what does it have to do with correlation?
If we have a player projected for 55 yards, and Underdog has his projection at 50 yards, we are essentially saying that Underdog is making a five-yard error in their projection. In the above example, we would be saying Underdog is making an error on every player. Why? Because the errors all exist for the same reason (the projected volume), and thus, they are correlated. Since we can’t observe this correlation the way we see direct correlation in action (like on a long pass), it is, in a way, hidden.
Importantly, this type of correlation is not (always) penalized in the payouts. Here’s another example:
Suppose you think Travis Kelce should project for a 30% target share and Rashee Rice should project for a 20% target share. If PrizePicks’ projections assume they’re both at 25%, then you’d want to choose more for Kelce and less for Rice. If you’re right about Kelce having the higher target share, both are good picks. Getting two picks correct from one prediction is pretty much the definition of correlation.
Conditional Probabilities and Correlation
If I asked you “What do you think the chances are that there will be flooding today?”, you might think “that’s an odd question, it rarely floods.” But what if you then looked out the window and saw that it was pouring rain? Now, what if you also remembered that it poured yesterday? Suddenly flooding would be pretty likely, right?
In this example, the raw probability of flooding was low, because flooding itself is rare. However, with the first condition, that it was pouring outside at the moment, the probability of flooding increased. This was, by definition, the conditional probability of flooding, given the fact that it was pouring outside. The conditional probability of flooding given that it also poured yesterday was even higher. This is exactly what conditional probability is — the probability of an event occurring, with the knowledge that some other event, the condition, will also or has also occurred.
Out of the hundred (thousands?) of pieces of content I’ve produced for FTN, one of my all-time favorites was a PrizePicks strategy video I did with the phenomenal Chris Meaney (see below). We walked through exactly how to think of conditional probabilities as it relates to correlated pick ‘em plays, but I’ll re-hash the argument here.
Recall the example of Mookie Betts and Freddie Freeman from earlier. Suppose on a given night that they each had fantasy point projections of 6.5 points. If I asked you if those were correlated, you would obviously say yes. However, if I asked you how correlated they were, would you even know where to begin when trying to respond? The answer is conditional probabilities. The probability of Betts getting more than 6.5 fantasy points, under the condition that Freeman does, is certainly higher than the raw probability that he goes over. How do we know this? The earlier example was a perfect illustration. Each player would have received five fantasy points for the double and two more for the run and RBI. Without the other doubling, they would have only had five fantasy points. Because of the other’s double, they each had seven.
Whenever you ask yourself how correlated two players or picks are, think of the conditional probabilities!
Why Strong Simulations Matter
Ironically, this entire article is devoted to helping you understand correlation, and now I’m about to tell you that with strong simulations, you don’t even have to. Notice, I keep saying strong simulations, instead of just simulations. This is because I believe most simulations in the industry do a poor job of reflecting real life outcomes — if you can’t reflect real life outcomes, how can you expect to reflect real-life correlations? You can’t.
However, with strong, well thought-out simulations, the effect of correlation, and the degree of correlation, is inherently captured. In another bout of irony, my Kelce and Rice example from the Indirect Correlation section earlier had a flaw to it… If I asked you for the conditional probability that Rice would finish below his Underdog projection given Kelce finishes above his Underdog projection, would you say it’s higher or lower than his raw probability of finishing below the projection? To answer this, you would have to weigh competing ideas — on the one hand, Kelce finishing above could mean that he dominated targets more than expected, but it could also mean that Kansas City was particularly effective through the air (or just had a ton of pass volume). The former would mean that they’re inversely correlated, as assumed in the earlier example. The latter would imply an element of positive correlation (correlated errors) that was ignored in the earlier example. The only way to answer whether they’re positively correlated or inversely correlated is through simulation.
When two events are independent of one another, the probability of both things occurring is the probability of the first multiplied by the probability of the second. For simplicity’s sake, let’s return to the Betts and Freeman example and assume that in a difficult matchup, both were only 50% likely to clear the 6.5-fantasy-point threshold. Then, if they were independent of one another, the chances of both clearing it would be 25% (50% x 50%). However, both would clear it around 30% of the time, maybe even more like 35% of the time due to their positive correlation. Whenever two picks hit together in the simulations more often than we’d expect to see if they were independent of one another, we know that they’re positively correlated. We can even answer the question of how correlated they are by comparing the difference between the rate that they simulate to hit together and the rate that we would otherwise expect.
Now that the DFS pick ‘em sites are penalizing direct correlation, it’s more important than ever to:
- Understand and identify where the hidden correlation is
- Answer the question of how correlated two picks are, not just whether they’re correlated to begin with
You may be ready to crush your class presentation on correlation now, but our new Pick ‘Em Tool can still help you print money.